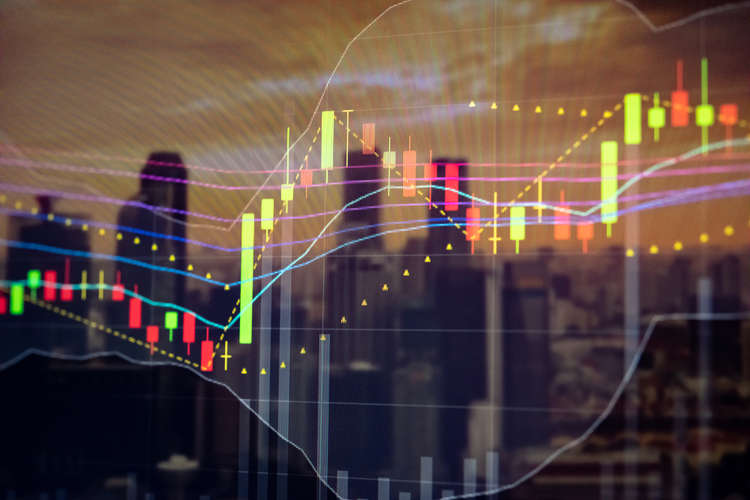
Motivation
Demand forecasting is the scientific backbone of several practical, business and policy uses cases in the energy sector. Forecasting may span over various time horizons, serving different, every time, mandates. In TRUST AI forecasting is addressed in a two fold way. First, at the building level and the short term, driven by real time data, where it can be the basis for several decisions, related to behaviour, system performance as well demand response schemes and dynamic pricing. And second at the country level and the mid term, driven by yearly data, where it supports key policy decisions for CO2 reduction and the evolution of the energy system.
Thus, the prime motivation is to pioneer explainable approaches based on genetic programming approaches in order to support and provide more confidence to the user (building level fiorecasting) and. Or policy maker (country level forecasting) when he is making operational decisions.
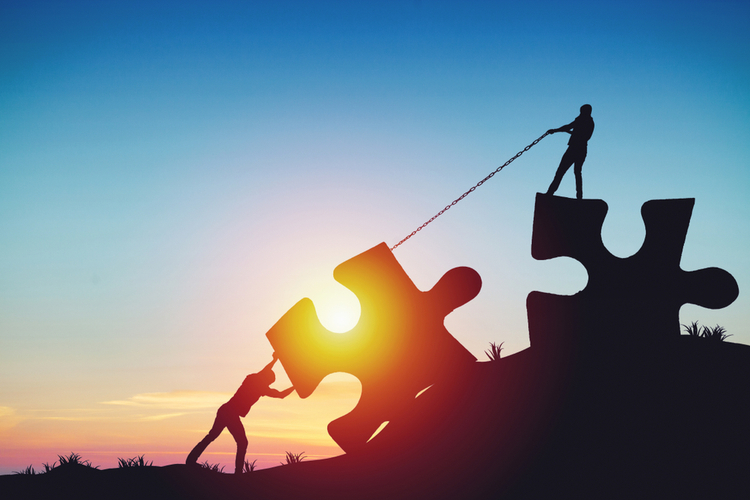
Challenge
Meeting the goals of energy transition beyond the carbon era will require significant advancements in material, process and data management technologies, as well as a better understanding and shaping of user behaviour into more eco-friendly profiles. For example, smart grids and in particular demand response schemes, hold a significant potential for the optimisation of the energy system, which is, however, currently lagging behind in deployment. A main reason for this is the important risk users are feeling there are exposed to. Tools to provide confidence and trust in the potential impact and benefits of the adoption of new pricing schemes can greatly add in the proliferation of such greatly beneficial schemes
We look forward to providing state of the art solutions, insight and advice in the two time horizons addressed (building and country) although a clear focus will be on the building level, in appreciation of the significant and short term challenges presented at that level.
Concise and explainable expressions, including feature importance and counterfactuals will allow users to understand what the impact of their action will be and come in better control of their built environment.
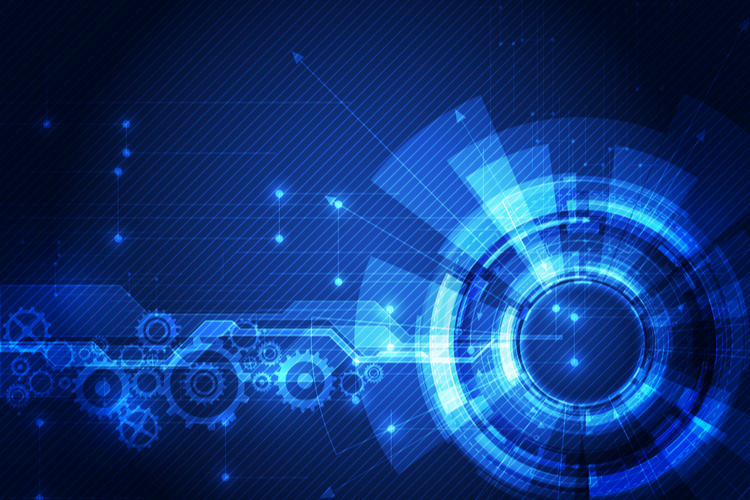
Approach
Real time, high resolution data management is the foundation of the data sourcing, for the building level use case. Neural networks can provided for high prediction accuracies but need to be complemented by insight, trust and an understanding of their results. To this extent, both counterfactual driven local explainability as well as global explainability via diverse surrogate models will be experimented upon in both sub cases.
The approach in TRUST AI will start from benchmarking genetic programming approaches against traditional LSTM and STS approaches used for the forecasting problem. This will help to establish whether there are any inherent performance issues with the explainable approaches pursued. Additionally, we look forward to explore feature importance and counterfactuals in order to provide tangible tools to building users and facility managers alike to support them in operational decisions.
Results
We have established for the first time in such a comprehensive manner the topics of XAI in the building energy arena. The publications sent out in quality journals (see respective page) in the area and the accumulation up until now (mid 2024) of 12 citations are clear manifestations of this.
We have validated that explainable approaches and related services, as offered by TRUST AI, can unleash radically new value; this value has been firmly analysed and some first conclusions have been drawn. Concisely, the key benefits reside in the explainable symbolic expressions that come at no expense of accuracy as well as in the support for feature importance and counterfactual investigations. Arguably, one will require further modelling for a significant number of buildings in order to shed light on the many possible and potential benefits that cannot otherwise surface.
We have developed energy specific integration requirements for TRUST AI. We have done this as a distinct functionality integration and have shown how this is accessed via specific technology platforms, commercial and open source alike.
We have highlighted the conditions for TRUST AI Energy sustainability and look forward to working in this direction in the coming period, with the aspiration to deliver to the community a sustainable TRUST AI for Energy resource.
The next day
We look forward to delivering an open source platform for the energy domain, labeled TRUST AI for Energy, centered around local (instance) and global (model) explainable approaches. We also aspire to integrate and support a LLM conversation with the models elaborated, something that we have recently started engaging with in TRUST AI and which would for the case of Energy unleash unique and new value.
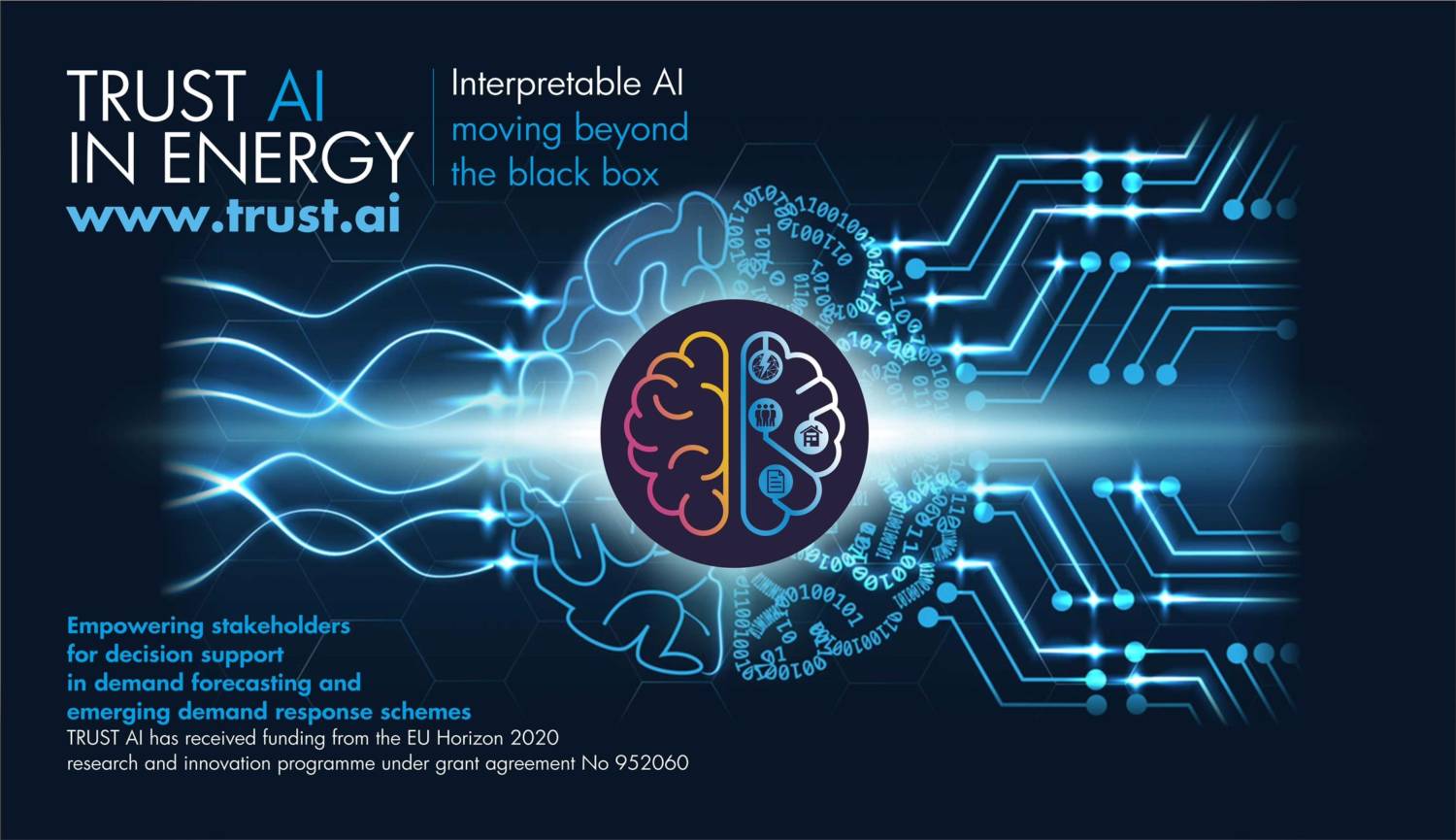